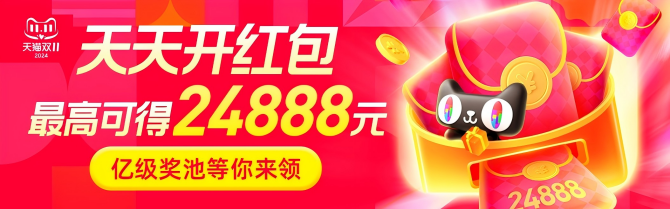
文章目录
- 1.文本识别数据集
-
- 1.1.Synthetic Chinese String Dataset
- 2.文本检测数据
-
- ICPR MWI 2018 挑战赛
- 2.1.Pascal VOC2007
- 2.2.MSRA Text Detection 500 Database (MSRA-TD500)
- 2.3.COCO-TEXT
- 2.4.Google FSNS(谷歌街景文本数据集)
- 2.5.Reading Chinese Text in the Wild(RCTW-17)
- 2.6.Chinese Text in the Wild(CTW)
- 2.7.中文数据集的自动合成
- 2.8.OCR数据集list
- 2.9.SynthText in the Wild dataset
- 3.扭曲文本
-
- 3.1.Total-Text
- 4.icdar
- reference:
1.文本识别数据集
1.1.Synthetic Chinese String Dataset
该数据集是中文识别数据集,包含360多万张训练图片,5824个字符,不过场景比较简单,图片是白底黑字。
下载地址:https://pan.baidu.com/s/1dFda6R3
图片,文字标签
2.文本检测数据
ICPR MWI 2018 挑战赛
大赛提供20000张图像作为数据集,其中50%作为训练集,50%作为测试集。主要由合成图像,产品描述,网络广告构成。该数据集数据量充分,中英文混合,涵盖数十种字体,字体大小不一,多种版式,背景复杂。文件大小为2GB。
https://tianchi.aliyun.com/competition/information.htm?raceId=231651&_is_login_redirect=true&accounttraceid=595a06c3-7530-4b8a-ad3d-40165e22dbfe
链接:https://pan.baidu.com/s/1zxXokAYsyVbfWP2dUPGrPw
提取码:z1bj
2.1.Pascal VOC2007
$ cd $FRCN/data
$ wget http://host.robots.ox.ac.uk/pascal/VOC/voc2007/VOCtrainval_06-Nov-2007.tar
$ wget http://host.robots.ox.ac.uk/pascal/VOC/voc2007/VOCtest_06-Nov-2007.tar
$ wget http://host.robots.ox.ac.uk/pascal/VOC/voc2007/VOCdevkit_08-Jun-2007.tar
$ wget http://host.robots.ox.ac.uk/pascal/VOC/voc2012/VOCtrainval_11-May-2012.tar
$ tar xvf VOCdevkit_08-Jun-2007.tar
$ tar xvf VOCtrainval_06-Nov-2007.tar
$ tar xvf VOCtest_06-Nov-2007.tar
$ ln -s VOCdevkit VOCdevkit2007 #create a softlink
链接:https://pan.baidu.com/s/1n3HSbDVZ-75SXC1PNC7bHA
提取码:8k9a
复制这段内容后打开百度网盘手机App,操作更方便哦
2.2.MSRA Text Detection 500 Database (MSRA-TD500)
MSRA文本检测500数据库(MSRA-TD500)包含500个自然图像,使用数据包相机从室内(办公室和商场)和室外(街道)场景拍摄,室内图像主要是标志,门板和警示牌,而室外图像主要是复杂背景下的导板和广告牌。图像的分辨率从1296×864到1920×1280不等。由于文本的多样性和图像背景的复杂性,数据集非常具有挑战性。文本可以是不同的语言(中文,英文或两者的混合),字体,大小,颜色和方向。
http://www.iapr-tc11.org/mediawiki/index.php/MSRA_Text_Detection_500_Database_%28MSRA-TD500%29
http://www.iapr-tc11.org/dataset/MSRA-TD500/MSRA-TD500.zip
0 0 749 860 47 105 -0.048040
1 1 728 919 16 44 -0.023252
2.3.COCO-TEXT
英文数据集,包括63686幅图像,173589个文本实例,包括手写版和打印版,清晰版和非清晰版。文件大小12.58GB,训练集:43686张,测试集:10000张,验证集:10000张。
下载地址:https://vision.cornell.edu/se3/coco-text-2/
COCO-Text API
The COCO-Text API assists in loading and parsing the annotations in COCO-Text. For details, see coco.py and also the coco_text_Demo ipython notebook.
getAnnIds Get ann ids that satisfy given filter conditions
getImgIds Get img ids that satisfy given filter conditions
loadAnns Load anns with the specified ids.
loadImgs Load imgs with the specified ids.
loadRes Load algorithm results and create API for accessing them.
The annotations are stored using the JSON file format. The annotations format has the following data structure:
{
“info” : info,
“imgs” : [image],
“anns” : [annotation]
}
info{
“version” : str,
“description” : str,
“author” : str,
“url” : str,
“date_created” : datetime
}
image{
“id” : int,
“file_name” : str,
“width” : int,
“height” : int,
“set” : str # ‘train’ or ‘val’
}
Each text instance annotation contains a series of fields, including an enclosing bounding box, category annotations, and transcription.
annotation{
“id” : int,
“image_id” : int,
“class” : str # ‘machine printed’ or ‘handwritten’ or ‘others’
“legibility” : str # ‘legible’ or ‘illegible’
“language” : str # ‘english’ or ‘not english’ or ‘na’
“area” : float,
“bbox” : [x,y,width,height],
“utf8_string” : str,
“polygon” : []
}
2.4.Google FSNS(谷歌街景文本数据集)
该数据集是从谷歌法国街景图片上获得的一百多万张街道名字标志,每一张包含同一街道标志牌的不同视角,图像大小为600*150,训练集1044868张,验证集16150张,测试集20404张。
下载地址:http://rrc.cvc.uab.es/?ch=6&com=downloads
2.5.Reading Chinese Text in the Wild(RCTW-17)
该数据集包含12263张图像,训练集8034张,测试集4229张,共11.4GB。大部分图像由手机相机拍摄,含有少量的屏幕截图,图像中包含中文文本与少量英文文本。图像分辨率大小不等。icdar2017rctw_train_v1.2
下载地址:http://rctw.vlrlab.net/dataset/
icdar2017rctw_train_v1.2
图片,坐标位置和文本
一串字一个方框
,,,,,,,,,""; ,,,,,,,,,""; …
例子:
390,902,1856,902,1856,1225,390,1225,0,“金氏眼镜”
1875,1170,2149,1170,2149,1245,1875,1245,0,“创于1989”
2054,1277,2190,1277,2190,1323,2054,1323,0,“城建店”
768,1648,987,1648,987,1714,768,1714,0,“金氏眼”
897,2152,988,2152,988,2182,897,2182,0,“金氏眼镜”
1457,2228,1575,2228,1575,2259,1457,2259,0,“金氏眼镜”
1858,2218,1966,2218,1966,2250,1858,2250,0,“金氏眼镜”
231,1853,308,1843,309,1885,230,1899,1,“谢#惠顾”
125,2270,180,2270,180,2288,125,2288,1,"###"
106,2297,160,2297,160,2316,106,2316,1,"###"
22,2363,82,2363,82,2383,22,2383,1,"###"
524,2511,837,2511,837,2554,524,2554,1,"###"
455,2456,921,2437,920,2478,455,2501,0,“欢迎光临”
396,2287,1079,2287,1079,2717,396,2717,0,“富士”
1159,2394,1361,2394,1361,2788,1159,2788,0,“壽司”
1434,2496,1682,2496,1682,2815,1434,2815,0,“屋”
2.6.Chinese Text in the Wild(CTW)
该数据集包含32285张图像,1018402个中文字符(来自于腾讯街景), 包含平面文本,凸起文本,城市文本,农村文本,低亮度文本,远处文本,部分遮挡文本。图像大小2048*2048,数据集大小为31GB。以(8:1:1)的比例将数据集分为训练集(25887张图像,812872个汉字),测试集(3269张图像,103519个汉字),验证集(3129张图像,103519个汉字)。
下载地址:https://ctwdataset.github.io/
https://share.weiyun.com/50hF1Cc
https://ctwdataset.github.io/tutorial/1-basics.html
图片,文本框的坐标
{annotations, [{adjusted_bbox, “attributes”: [“distorted”, “raised”], is_chinese,polygon,}], file_name, height, ignore, width, image_id}
一个字一个方框
Training set annotation format
All .jsonl annotation files (e.g. …/data/annotations/train.jsonl) are UTF-8 encoded JSON Lines, each line is corresponding to the annotation of one image.
The data struct for each of the annotations in training set (and validation set) is described below.
annotation (corresponding to one line in .jsonl):
{
image_id: str,
file_name: str,
width: int,
height: int,
annotations: [sentence_0, sentence_1, sentence_2, …], # MUST NOT be empty
ignore: [ignore_0, ignore_1, ignore_2, …], # MAY be an empty list
}
sentence:
[instance_0, instance_1, instance_2, …] # MUST NOT be empty
instance:
{
polygon: [[x0, y0], [x1, y1], [x2, y2], [x3, y3]], # x, y are floating-point numbers
text: str, # the length of the text MUST be exactly 1
is_chinese: bool,
attributes: [attr_0, attr_1, attr_2, …], # MAY be an empty list
adjusted_bbox: [xmin, ymin, w, h], # x, y, w, h are floating-point numbers
}
attr:
“occluded” | “bgcomplex” | “distorted” | “raised” | “wordart” | “handwritten”
ignore:
{
polygon: [[x0, y0], [x1, y1], [x2, y2], [x3, y3]],
bbox: [xmin, ymin, w, h],
]
2.7.中文数据集的自动合成
github地址:https://github.com/JarveeLee/SynthText_Chinese_version
2.8.OCR数据集list
github地址:https://github.com/xylcbd/ocr-open-dataset
2.9.SynthText in the Wild dataset
This dataset consists of 8 million images covering 90k English words, and includes the training, validation and test splits used in our work.
该数据集包含8百万张图片,涵盖9万个英文单词。出自牛津大学。
下载地址:http://www.robots.ox.ac.uk/~vgg/data/scenetext/
3.扭曲文本
3.1.Total-Text
该数据集共1555张图像,11459文本行,包含水平文本,倾斜文本,弯曲文本。文件大小441MB。大部分为英文文本,少量中文文本。训练集:1255张 测试集:300
下载地址:http://www.cs-chan.com/source/ICDAR2017/totaltext.zip
https://github.com/cs-chan/Total-Text-Dataset
4.icdar
https://rrc.cvc.uab.es/?ch=8&com=downloads
4.1.DocVQA-2020
4.1.1.overview
4.1.2.tasks
4.1.3.downloads
4.1.4.results
4.1.5.my methods
4.1.6.organizers
4.2.ST-VOA-2019
4.2.1.overview
4.2.2.tasks
4.2.3.downloads
4.2.4.results
4.2.5.my methods
4.2.6.organizers
4.3.MLT-2019
overview
tasks
downloads
results
my methods
organizers
4.4.LSVT-2019
4.5.ArT-2019
4.6.SROIE-2019
4.7.ReCTS-2019
4.8.COCO-Text-2017
4.9.DeTEXT-2017
4.10.DOST-2017
4.11.FSNS-2017
4.12.MLT-2017
4.13.IEHHR-2017
4.14.Incidental Scene Text-2015
4.15.Text in Videos-2013-2015
4.16.Focused Scene Text-2013-2015
4.17.Born-Digital Images(Web and Email)-2011-2015
4.17.1.overview
Overview – Born-Digital Images (Web and Email)
Images are frequently used in electronic documents (Web and email) to embed textual information. The use of images as text carriers stems from a number of needs. For example images are used in order to beautify (e.g. titles, headings etc), to attract attention (e.g. advertisements), to hide information (e.g. images in spam emails used to avoid text-based filtering), even to tell a human apart from a computer (CAPTCHA tests).
Automatically extracting text from born-digital images is therefore an interesting prospect as it would provide the enabling technology for a number of applications such as improved indexing and retrieval of Web content, enhanced content accessibility, content filtering (e.g. advertisements or spam emails) etc.
While born-digital text images are on the surface very similar to real scene text images (both feature text in complex colour settings) at the same time they are distinctly different. Born-digital images are inherently low-resolution (made to be transmitted online and displayed on a screen) and text is digitally created on the image; scene text images on the other hand are high-resolution camera captured ones. While born-digital images might suffer from compression artefacts and severe anti-aliasing they do not share the illumination and geometrical problems of real-scene images. Therefore it is not necessarily true that methods developed for one domain would work in the other.
In 2011 we set out to find out the state of the art in Text Extraction in both domains (born-digital images and real scene). We received 24 submissions over three different tasks in the born-digital Challenge, 10 during the competition run and 14 more over the following year, after the competition was opened in a continuous mode in October 2011.
Given the strong interest displayed by the community, and the fact that there is still a large margin for improvement, in the ICDAR 2013 edition we revisited the tasks of localisation, segmentation and recognition and invited further submissions on an updated and even more challenging dataset. We received 13 submissions during the 2013 edition and the year following it, when the competition was opened in a continuous mode.
For the 2015 edition, we are introducing a new task: End-to-End, referring to text localisation and recognition in a single go at the word level. The rest of the tasks remain open in a continuous mode, unchanged form the 2013 edition. See details in the Tasks page.
The results from the past ICDAR competitions can be found in the ICDAR proceedings [1, 2].
D.Karatzas, F. Shafait, S. Uchida, M. Iwamura, L. Gomez, S. Robles, J. Mas, D. Fernandez, J. Almazan, L.P. de las Heras , “ICDAR 2013 Robust Reading Competition”, In Proc. 12th International Conference of Document Analysis and Recognition, 2013, IEEE CPS, pp. 1115-1124. [pdf] [poster] [presentation][pdf] [poster] [presentation]
D. Karatzas, S. Robles Mestre, J. Mas, F. Nourbakhsh, P. Pratim Roy , “ICDAR 2011 Robust Reading Competition – Challenge 1: Reading Text in Born-Digital Images (Web and Email)”, In Proc. 11th International Conference of Document Analysis and Recognition, 2011, IEEE CPS, pp. 1485-1490. [pdf] [presentation]
[pdf] [presentation]
4.17.2.tasks
Tasks – Born-Digital Images (Web and Email)
The Challenge is set up around four tasks:
Text Localization, where the objective is to obtain a rough estimation of the text areas in the image, in terms of bounding boxes that correspond to parts of text (words or text lines).
Text Segmentation, where the objective is the pixel level separation of text from the background.
Word Recognition, where the locations (bounding boxes) of words in the image are assumed to be known and the corresponding text transcriptions are sought.
End-to-End, where the objective is to localise and recognise all words in the image in a single step.
For the 2015 edition, the focus is solely on task T1.4 “End-to-End”. The rest of the tasks are open for submissions but will not be included / analysed in the ICDAR 2015 report.
A training set of 410 images (containing 3564 words) is provided through the downloads section. The training set is common for all three tasks, although different ground truth data is provided for each of them.
All images are provided as PNG files and the text files are ASCII files with CR/LF new line endings.
4.17.2.1.Task 1.1: Text Localization
For the text localization task we provide bounding boxes of words for each of the images. The ground truth is given as separate text files (one per image) where each line specifies the coordinates of one word’s bounding box and its transcription in a comma separated format (see Figure 1).
For the text localization task the ground truth data is provided in terms of word bounding boxes. For each image in the training set a separate ASCII text file will be provided, following the naming convention:
gt_[image name].txt
The text files are comma separated files, where each line will corresponds to one word in the image and gives its bounding box coordinates and its transcription in the format:
left, top, right, bottom, “transcription”
Please note that the escape character () is used for double quotes and backslashes (see for example img_4 in Figure 1).
The authors will be required to automatically localise the text in the images and return bounding boxes. The results will have to be submitted in separate text files for each image, with each line corresponding to a bounding box (comma separated values) as per the above format. A single compressed (zip or rar) file should be submitted containing all the result files. In the case that your method fails to produce any results for an image, you can either include an empty result file or no file at all.
The evaluation of the results will be based on the algorithm of Wolf et al [1] which in turn is an improvement on the algorithms used in the robust reading competitions in previous ICDAR instalments.
4.17.2.2.Task 1.2: Text Segmentation
For the text segmentation task, the ground truth data is provided in the form of colour-coded PNG images following the naming convention:
gt_[image name].png
In the ground truth images, white pixels should be interpreted as background pixels, while non-white pixels as text (see Figure 2). The non-white pixels are colour coded, so that each atom in the image is shown in the same colour. An atom is defined in accordance to [2] as the minimum set of connected components that can be assigned a semantic interpretation. So atoms might comprise single components that correspond to one or multiple (e.g. in the case of cursive text) characters, or they might comprise multiple components that correspond to one (e.g. letters “i”, “j”, the letters of the IBM logo) or multiple characters.
The authors will be asked to automatically segment the test images and submit their segmentation result as a series of bi-level images, following the same format. A single compressed (zip or rar) file should be submitted containing all the result files. In the case that your method fails to produce any results for an image, you can either include an empty result file or no file at all.
Evaluation will be primarily based on the methodology proposed by the organisers in the paper [2], while a typical precision / recall measurement will also be provided for consistency, in the same fashion as [3].
4.17.2.3.Task 1.3: Word Recognition
For the word recognition task, we provide all the words in our dataset with 3 characters or more in separate image files, along with the corresponding ground-truth transcription (See Figure 2 for examples). The transcription of all words is provided in a SINGLE text file for the whole collection. Each line in the ground truth file has the following format:
[image name], “transcription”
An example is given in figure 3. Please note that the escape character () is used for double quotes and backslashes (see for example the transcriptions of 15.png and 20.png in Figure 3).
For testing we will provide the images of about 400 words and we will ask for the transcription of each image. A single transcription per image will be requested. The authors should return all result transcriptions in a single text file of the same format as the ground truth.
For the evaluation we will calculate the edit distance between the submitted image and the ground truth transcription. Equal weights will be set for all edit operations. The best performing method will be the one with the smallest total edit distance.
Note that words are cut-out with a frame of 4 pixels around them (instead of the tight bounding box), in order to preserve the immediate context. This is usual practice to facilitate processing (see for example the MNIST character dataset).
4.17.2.4.Task 1.4: End to End
Ground truth is provided for each image of the training set that comprises the bounding quadrilateral of each word as well as the transcription of the word. The ground truth is the same as for Task 1.1. One- or two-character words as well as words deemed unreadable are annotated in the dataset as “do not care” following the ground truthing protocol (to be made public).
Vocabularies
Apart from the transcription and location ground truth we provide a generic vocabulary of about 90k words, a vocabulary of all words in the training set and per-image vocabularies of 100 words comprising all words in the corresponding image as well as distractor words selected from the rest of the training set vocabulary, following the setup of Wang et al [4]. Authors are free to incorporate other vocabularies / text corpuses during training to enhance their language models, in which case they will be requested to indicate so at submission time to facilitate the analysis of results.
All vocabularies provided contain words of 3 characters or longer comprising only letters.
Vocabularies do not contain alphanumeric structures that correspond to prices, URLs, times, dates, emails etc. Such structures, when deemed readable, are tagged in the images and an end-to-end method should be able to recognise them, although the vocabularies provided do not inlcude them explicitly.
Words were stripped by any preceding or trailing symbols and punctuation marks before they were added in the vocabulary. Words that still contained any symbols and puctuation marks (with the exception of hyphens) were filtered as well. So for example “e-mail” is a valid vocabulary entry, while “rrc.cvc.uab.es” is a non-word and is not included.
Submission Stage
For the test phase, we will provide a set of test images along with three specific lists of words for each test image that comprise:
Strongly Contextualised: per-image vocabularies of 100 words including all words (3 characters or longer, only letters) that appear in the image as well as a number of distractor words chosen at random from the same subset test following the setup of Wang et al [4],
Weakly Contextualised: all words (3 characters or longer, only letters) that appear in the entire test set, and
Generic: any vocabulary can be used, a 90k word vocabulary is provided
For each of the above variants, participants can make use of the corresponding vocabulary given to guide the end-to-end word detection and recognition process.
Participants will be able to submit end-to-end results for these variants in a single submission step. Variant (1) will be obligatory, while variants (2) and (3) optional.
Along with the submission of results, participants will have the option to submit the corresponding executable binary file (Windows, Linux or Mac executable). This optional binary file can be added to the submission at a later time (there is no need to delay the submission of results). The executable of the method will be used over a hidden test subset to further analyse the method and provide insight to the authors. The ownership of the file remains with the authors, and the organisers of the competition will keep the executable private and will not make use of the executable in any way unrelated to the competition. The executable should be:
Windows, linux, Mac executable
Compiled for single core architectures
Have no external dependencies (statically linked, or all libraries given)
Command line, no graphical interface
In Parameters: vocabulary filename (e.g. images/img.txt), image filename (e.g. images/img.png)
Output: text file of results for the image same format as the submission called out.txt
4.17.2.5.Evaluation
The evaluation protocol proposed by Wang 2011 [4] will be used which considers a detection as a match if it overlaps a ground truth bounding box by more than 50% (as in [5]) and the words match, ignoring the case. Detecting or missing words marked as “do not care” will not affect (positively or negatively) the results. Any detections overlapping more than 50% with “do not care” ground truth regions will be discarded from the submitted results before evaluation takes place, and evaluation will not take into account ground truth regions marked as “do not care”.
References
C. Wolf and J.M. Jolion, “Object Count / Area Graphs for the Evaluation of Object Detection and Segmentation Algorithms”, International Journal of Document Analysis, vol. 8, no. 4, pp. 280-296, 2006.
A. Clavelli, D. Karatzas, and J. Llados, “A Framework for the Assessment of Text Extraction Algorithms on Complex Colour Images”, in Proceedings of the 9th IAPR Workshop on Document Analysis Systems, Boston, MA, 2010, pp. 19-28.
K. Ntirogiannis, B. Gatos, and I. Pratikakis, “An Objective Methodology for Document Image Binarization Techniques”, in Proceedings of the 8th International Workshop on Document Analysis Systems, Nara, Japan, 2008, pp. 217-224
K. Wang, B. Babenko, and S. Belongie, “End-to-end scene text recognition”, in Computer Vision (ICCV), 2011 IEEE International Conference on (pp. 1457-1464), IEEE, November 2011
M. Everingham, S. A. Eslami, L. Van Gool, C. K. Williams, J. Winn, and A. Zisserman, (2014). The pascal visual object classes challenge: A retrospective. International Journal of Computer Vision, 111(1), 98-136.
4.17.3.downloads
5.Downloads – Born-Digital Images (Web and Email)
Download below the training dataset and associated ground truth information for each of the Tasks. Task 1.4 is new for the 2015 edition.
5.1.Task 1.1: Text Localization (2013 edition)
5.1.1.Training set
Training set Images (33Mb). – 410 images that comprise the training dataset.
Training Set Text Localization Ground Truth (88Kb). – 410 Text files (one per image) as explained in the “Tasks” section.
5.1.2.Test Set
Test Set Images (5.6Mb). – 141 images that comprise the test set for tasks 1.1, 1.2 and 1.4. You can submit your results for this Task over the images of the test set through the My Methods section.
Test Set Ground Truth (40Kb). – 141 text files with text localisation bounding boxes for the images of the test set.
5.2.Task 1.2: Text Segmentation (2013 edition)
5.2.1.Training Set
Training Set Images (33Mb). – 410 images that comprise the training dataset. This is the same dataset as for Task 1.1.
Training Set Text Segmentation Ground Truth (943Kb). – 410 colour coded images as explained in the “Tasks” section.
5.2.2.Test Set
Test Set Images (5.6Mb). – 141 images that comprise the test set for tasks 1.1, 1.2 and 1.4. You can submit your results for this Task over the images of the test set through the My Methods section.
Test Set Ground Truth (377Kb). – 141 colour coded image corresponding to the images of the test set. Each colour marks a different atom – white is background.
5.3.Task 1.3: Word Recognition (2013 edition)
5.3.1.Training Set
Training Set Word Images and Ground Truth (12Mb). – 3564 images of words cut from the original images and a single text file with the ground truth transcription of all images as specified in the “Tasks” section.
5.3.2.Test Set
Test Set Word Images (4.6Mb). – 1439 images that comprise the word recognition test set. You can submit your results for this Task over the images of the test set through the My Methods section.
Test Set Ground Truth (34Kb). – A single text file with the transcriptions of the 1439 images of the test set. Each line corresponds to an image of the test set.
5.4.Task 1.4: End to End (2015 edition)
5.4.1.Training Set
Training set Images (33MB). – 410 images that comprise the training dataset.
Training Set Text Localization and Transcription Ground Truth (118KB). – 410 Text files (one per image). Each line corresponds to one word and comprises the coordinates of the four corners of the bounding box given in a clockwise order in a comma separated list, and the transcription following the eighth comma.
Training vocabularies per image (214KB). – Vocabularies of 100 words per image, comprising the words appearing in the image plus distractors.
Training set vocabulary (12KB). – Vocabulary of all words (words of 3 characters or longer comprising only letters) appearing in the training set.
5.4.2.Test Set
Test Set Images (5.6Mb). – 141 images that comprise the test set for tasks 1.1, 1.2 and 1.4. You can submit your results for this Task over the images of the test set through the My Methods section.
Test vocabularies per image (75KB). – Vocabularies of 100 words per image, comprising the words appearing in the image plus distractors.
Test set vocabulary (6KB). – Vocabulary of all words (words of 3 characters or longer comprising only letters) appearing in the test set.
5.4.3.Other
Generic Vocabulary (796KB).- A vocabulary of about 90k words derived from the dataset publicly available here. Please consult [1,2] for further information as well as the disclaimer in the vocabulary file itself.
5.5.Sample MatLAB Code
Sample MatLAB Code (1Mb). – Sample code in MatLAB illustrating how to read in the training images and ground truth and how to output results for the tasks 1.1, 1.2 and 1.3.
5.6.Terms of Use
The “Born-Digital Images” dataset and corresponding annotations are licensed under a Creative Commons Attribution 4.0 License.
5.7.References
M. Jaderberg, K. Simonyan, A. Vedaldi, and A. Zisserman, “Synthetic data and artificial neural networks for natural scene text recognition”, arXiv preprint arXiv:1406.2227, 2014
M. Jaderberg, K. Simonyan, A. Vedaldi, and A. Zisserman, “Reading Text in the Wild with Convolutional Neural Networks”, arXiv preprint arXiv:1412.1842, 2014
5.7.1.results
5.7.2.my methods
5.7.3.organizers
reference:
https://blog.csdn.net/qq_14845119/article/details/105023984#comments